Camera for traffic light detection
- Timofey Uvarov
- Apr 14
- 3 min read
Updated: Apr 29
Flicker mitigated hardware solution for detecting LED and conventional traffic light signals.
Autonomous vision systems face significant challenges, especially in accurately detecting and interpreting traffic signals under varied environmental conditions. Traditional camera systems often struggle with accurately capturing LED traffic lights due to inherent flickering caused by their operational frequency (typically 50-60 Hz). This flicker can cause critical detection errors, showing traffic lights as blinking, entirely off, or falsely colored, posing significant safety risks.
Addressing this issue, a specialized AI-trained camera has been developed explicitly for reliable traffic light detection. This camera has been meticulously engineered to overcome issues arising from LED flicker and traditional incandescent lights, ensuring consistent and reliable detection both day and night.
Understanding the Challenge: LED Flicker
LED traffic lights cycle between on and off states at high frequencies. Standard automotive cameras operating around 30 fps occasionally capture LEDs during their off states, resulting in flickering or inaccurate color detection. This misrepresentation can lead autonomous systems to interpret signals incorrectly, such as identifying a functioning red light as faulty or misinterpreting its color due to partial saturation.
To understand the flicker the video below is very helpful:
Traditional HDR sensors, which utilize multiple exposures (T1, T2, T3) or split photodiode architecture (T1,T2) , compound this issue, as the final image may unpredictably capture LED states, leading to inconsistent results. To combat this, sensor manufacturers introduced "super exposure" technology that captures the entire LED flicker cycle in a single, extended exposure (approximately 10-11 milliseconds), thereby mitigating flicker-related issues. However, this method often results in oversaturation due to the prolonged exposure period.
Engineering the Solution: A Specialized Approach
This specialized camera system utilizes a fixed exposure time of approximately 11 milliseconds. To manage potential oversaturation from this prolonged exposure, the camera incorporates neutral density (ND) filters—effectively multiple layers of sunglasses—maintaining optimal exposure regardless of ambient light conditions. Remarkably, this approach remains effective even at night.
Lab experiments guided this configuration by systematically adjusting ND filters and lens apertures to identify the optimal balance for accurate color reproduction and exposure management. Results showed superior performance when using lenses with smaller f-numbers combined with ND filters, significantly reducing halo effects and enhancing LED color fidelity.
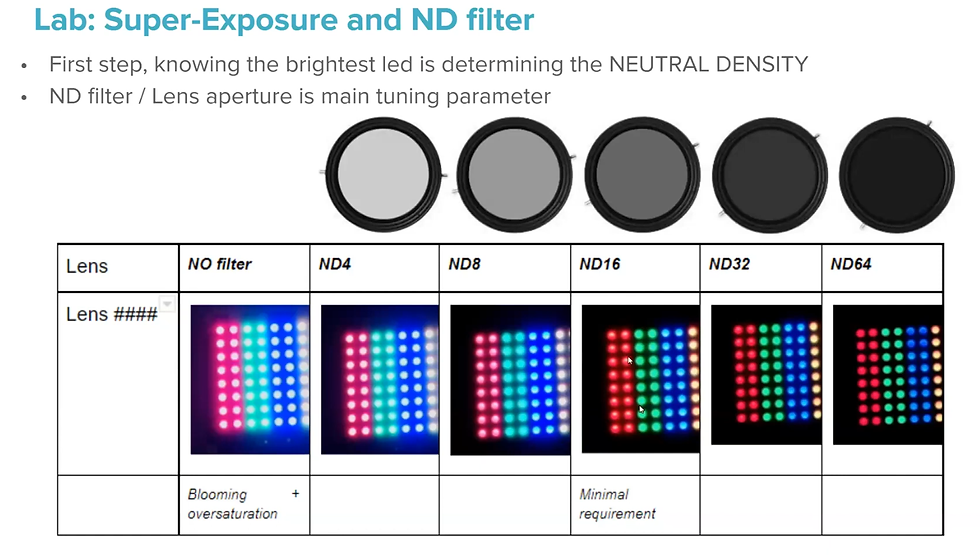
Advanced Tuning Techniques
Distinct from conventional cameras tuned for broad scene detection (objects, vehicles, lanes), this specialized camera undergoes tuning focusing on three core areas:
Exposure Control: Fixed integration time necessitates meticulous management of lens aperture and sensor gain to avoid oversaturation and ensure visibility of both bright and dim signals.
Color Accuracy and White Balance: Unlike traditional systems adjusting colors based on scene composition, this camera employs fixed white balance settings (D65), crucial for distinguishing closely related colors, such as red and yellow signals, solely differentiated by green channel intensity.
Tone Mapping and Gamma Adjustments: Fine-tuning gamma settings and sharpening algorithms significantly improved visibility and reduced halo effects, ensuring clear delineation of traffic signals.
Left: Traditional Camera, Right - Traffic light camera
Enhancing Night Vision Capabilities
Autonomous vision systems must perform reliably at night and under low-visibility conditions. Effective night vision involves enhancing sensitivity, reducing noise, and carefully managing exposure to maintain clear visibility of crucial signals. Specialized sensors and advanced image processing algorithms are utilized to improve low-light performance significantly.
Modern vision systems deploy techniques such as multi-exposure fusion and noise reduction algorithms, enabling cameras to maintain visibility and reliability across varying environmental conditions. The combination of these techniques ensures that traffic lights remain consistently detectable, whether illuminated brightly during the day or dimly at night.
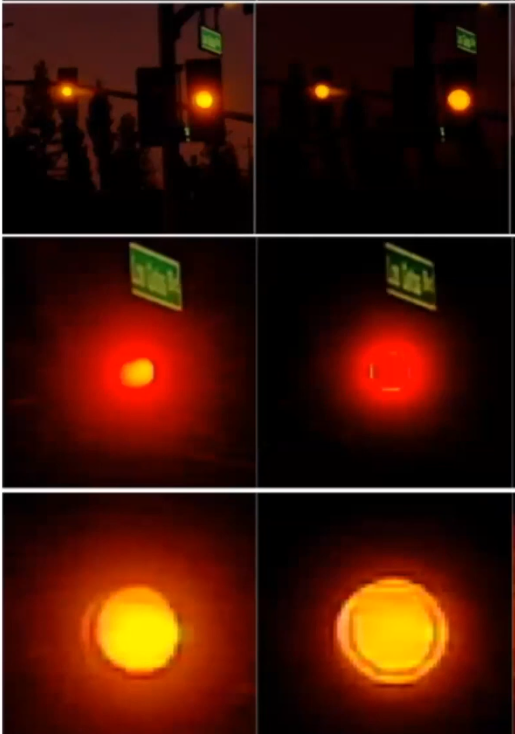
Real-world Performance
Deployment of this specialized camera demonstrated marked improvements in operational safety. In practical tests across various urban environments, including challenging scenarios with intense backlighting (such as the sun directly behind traffic signals), the camera reliably identified traffic lights without flicker or misrepresentation.
Real-world validation further confirmed this success. Following deployment and tuning, incidents involving traffic signal misinterpretation were substantially reduced, significantly enhancing overall vehicle safety.
Lets show some captures made with our traffic light camera by Pony.ai.
Below images were captured near mt. View Caltrain station:
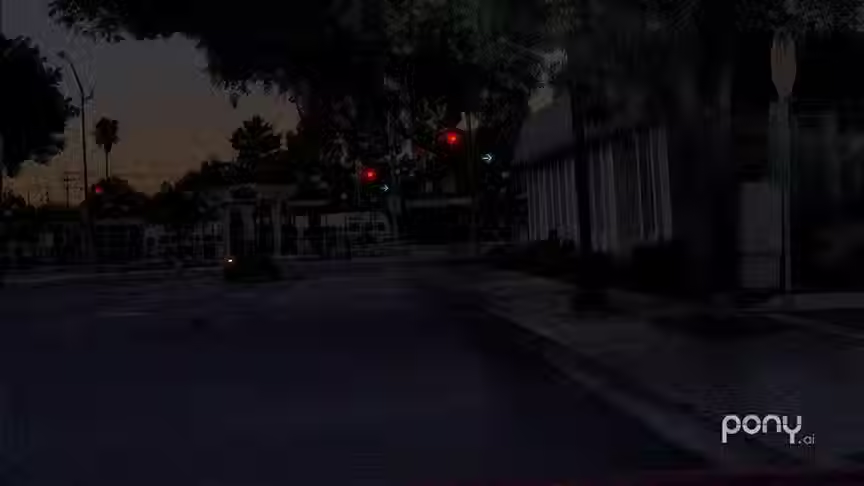
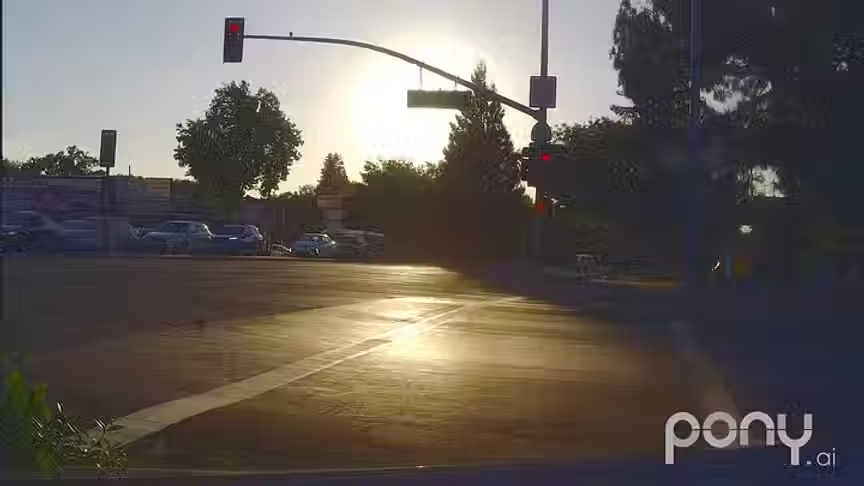
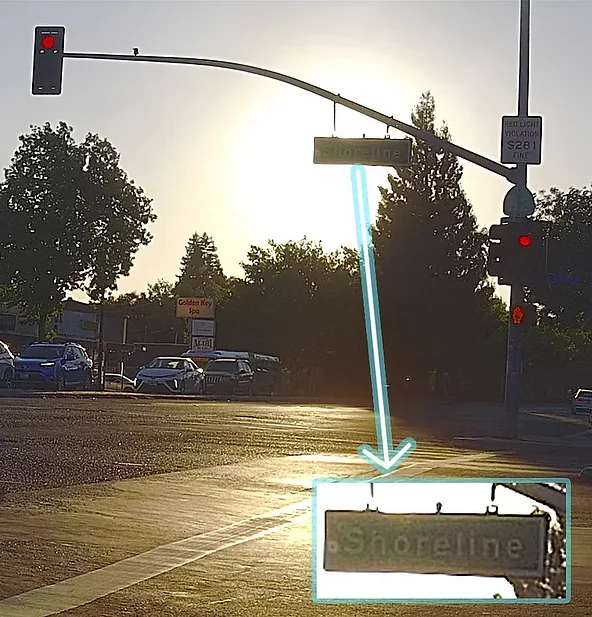
Implications and Future Applications
This specialized camera technology significantly enhances driving safety and reliability. Given the proliferation of LED traffic lights globally, widespread adoption of similar technologies by other autonomous vehicle developers appears necessary. Currently, many autonomous systems rely on general-purpose cameras or combined object-traffic detection systems, which may compromise safety in complex urban traffic environments.
In conclusion, specialized camera technology represents an essential advancement in autonomous vehicle perception, significantly improving the reliability and safety of traffic signal detection in diverse and challenging operational scenarios.
Explore how specialized camera technology solves critical challenges in traffic light detection for safer autonomous driving—day and night in the video below: